Machine Learning to Advance all Scientific Fields
Dr. Ofir Lindenbaum explores the field of machine learning and develops algorithms that can improve scientific research in almost every area – from the discovery of new cancer drugs to predicting floods
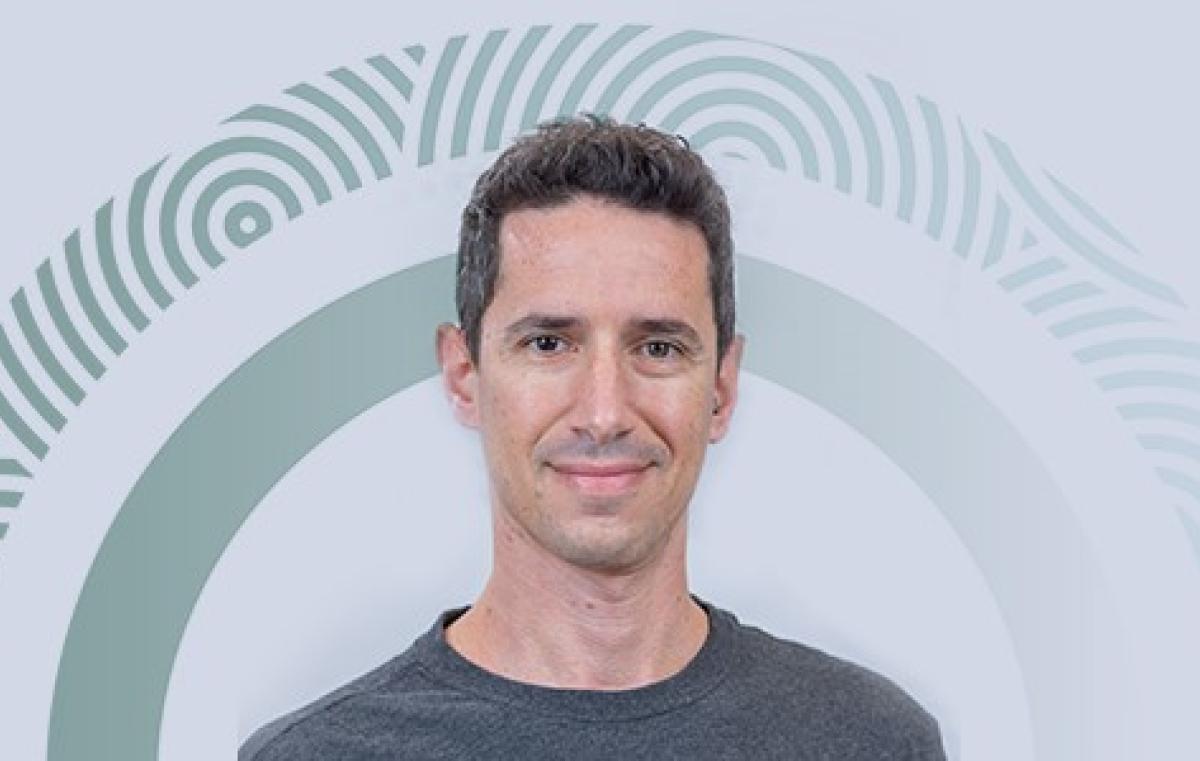
In recent years, there has been a marked improvement in the treatment of cancer. Scientific breakthroughs have led to innovative therapies, such as biological therapy, which is based on the modification of genes that affect the immune system's response to tumors. But even in this area, there is room for significant improvement. "Machine learning will allow us to find dozens, if not hundreds, of other genes of this type that are currently hidden," says Dr. Ofir Lindenbaum of the Faculty of Engineering. “Locating them will lead to the development of new, personalized, and much more effective drugs than the biological and, of course, the drugs that we have today.”
Lindenbaum specializes in machine learning. “At the outset of the research, I was involved in signal processing, and later, I shifted towards machine learning, where the question preoccupying me all along the way was how to extract from observable data the latent scientific information that interests us,” he says. “During my doctorate, I worked on integrating multi-view information obtained simultaneously from different measuring devices, such as audio and images from x-ray and MRI instruments. My goal was to learn how to use machine learning to find a fused representation that better characterized the latent information of the system of interest.”
During postdoctoral research in applied mathematics at Yale University, he recalls: “I dealt mainly with biological and medical data. My work at Yale was mainly with researchers from the faculties of medicine and biology, which would raise a scientific question and try to solve it by collecting high dimensional measurements, such as genetic information of cells or medical imaging” he relates. “My job was to develop tools that assist, via automation made possible by machine learning, to find patterns and variables that affect medical problems. I was at Yale for three and a half years, although the last year I was in Israel, due to COVID-19 – which enabled computational people like myself to work remotely.”
He arrived at BIU’s Faculty of Engineering in October 2021 and joined the Data Science track. He set up his group, Machine Learning for Scientific Discovery, which works on developing machine learning tools that will automatically assist in researching scientific questions. “My group is very diverse,” he says. “We are all involved in developing tools that will help with practical scientific problems – but in different areas. My lab is researching problems related to psychology, biology, and neuroscience. One collaboration with Dr. Vadim Axelrod of the Gonda Multidisciplinary Brain Research Center is engaged in predicting the people’s consciousness in a vegetative state using measurements called fMRI. Another cooperative project, with Prof. Sharon Ganot of the Faculty of Engineering, deals with signal processing. A third research study, with Dr. Yonatan Ganot of the Department of Geography, focuses on predicting urban floods.”
Concurrently, Dr. Lindenbaum has opened a new master’s degree course that deals with unsupervised learning. “Unsupervised learning is learning that does not require human tagging. In machine learning, much of the research is based on tagged examples, that is, those that require human labeling to allow training of machine learning models. For example images we know have a person’s facial characteristics, or audio clips, in which someone says a certain word,” he explains. “In contrast, models that don’t require tagging look for patterns within databases that sometimes even a person cannot. For example a search for genes that affect a certain medical problem in a table with hundreds of thousands of genes. It is difficult for humans to find patterns in such data, especially when the table is not organized. Another example of this cooperative effort can be found in a study I am conducting in cooperation with Dr. Yonatan Ganot that deals with flood prediction. Our goal in this project is to give a real-time alert to residents, in Tel Aviv or in other Gush Dan cities, for example, to prevent flooding of vehicles or basements where people reside. In this project, we measure data from the meteorological service and attach it to residents’ reports to give a forecast in time. This is the model-building phase, and it is based on tagged examples. But in real time, we would like to base it on untagged examples, which can predict the flood even before the report.”
Dr. Lindenbaum works with graduate students on several machine learning projects in his lab. “My dream is to be able to develop tools that can be used in any scientific field: that biologists, doctors, and geophysicists, for example, will use the tools that we developed, and that will help them to make new discoveries, which cannot be made with the tools they have today. I see myself dealing with this in the future, changing the way scientists work, improving and advancing science, and enabling it to make new discoveries.”